在本周二的阿姆斯特丹的思科全球网络会议(Cisco Live)上,企业网络巨头思科宣布与英伟达( Nvidia)合作推出一系列专为时下最受关注的热门词(AI/ML:人工智能/机器学习)量身定制的硬件和软件平台。
在本周二的阿姆斯特丹的思科全球网络会议(Cisco Live)上,企业网络巨头思科宣布与英伟达( Nvidia)合作推出一系列专为时下最受关注的热门词(AI/ML:人工智能/机器学习)量身定制的硬件和软件平台。
A key focus of the collaboration is making AI systems easier to deploy and manage using standard Ethernet, something we're sure all those who've gone through trouble getting their CCNA and/or CCNP certificates will appreciate.
两家合作的一个重点是使得用标准以太网部署和管理人工智能系统更加容易一些,相信那些费尽周折考 CCNA 和/或 CCNP 证书的人对此会深有体会。
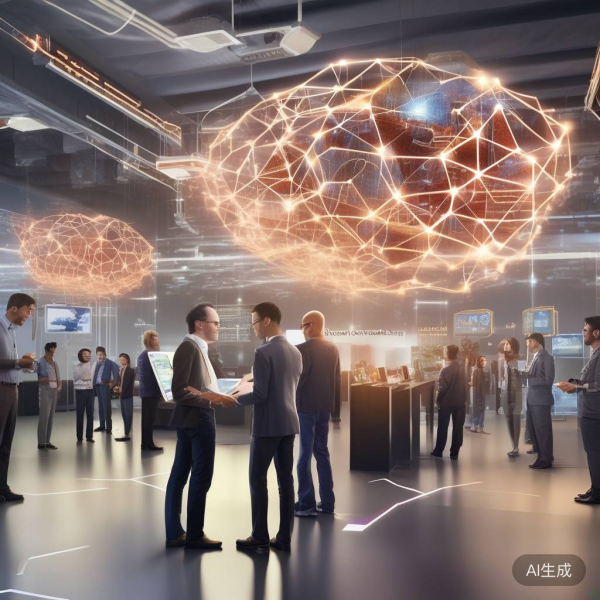
While the GPUs that power AI clusters tend to dominate the conversation, the high-performance, low-latency networks required to support can be quite complex. While it's true that modern GPU nodes benefit heavily from speedy 200Gb/s, 400Gb/s, and soon 800Gb/s networking, this is only part of the equation, particularly when it comes to training. Because these workloads often have to be distributed across multiple servers containing four or eight GPUs, any additional latency can lead to extended training times.
GPU为人工智能集群提供动力,也往往是讨论的焦点,但支持人工智能集群所需的高性能、低延迟网络可能相当复杂。现代 GPU 节点确实在很大程度上受益于 200Gb/s、400Gb/s 以及即将到来的 800Gb/s 高速网络,但这只是部分因素,尤其是在训练模型时。因为这些工作负载通常需要分布在包含四个或八个 GPU 的多台服务器上,任何额外的延迟都会导致训练时间的延长。
Because of this, Nvidia's InfiniBand continues to dominate AI networking deployments. In a recent interview with Dell'Oro Group's enterprise analyst Sameh Boujelbene estimated that about 90 percent of deployments are using Nvidia/Mellanox's InfiniBand — not Ethernet.
因此,Nvidia 的 InfiniBand 仍然在人工智能网络部署中占据主导地位。Dell'Oro Group 的企业分析师 Sameh Boujelbene 最近在接受采访时估计,约 90% 的部署使用的是 Nvidia/Mellanox 的 InfiniBand,而不是以太网。
That's not to say Ethernet isn't gaining traction. Emerging technologies, like smartNICs and AI-optimized switch ASICs with deep packet buffers have helped to curb packet loss, making Ethernet at least behave more like InfiniBand.
这并不是说以太网没有受到重视。一些新兴技术(例如带有深度数据包缓冲区的智能网卡(smartNIC)和人工智能优化交换机专用集成电路(ASIC)有助于抑制数据包丢失)使以太网至少可以更像 InfiniBand 一样运行。
For instance, Cisco's Silicon One G200 switch ASIC, which we looked at last summer, boasts a number of features beneficial to AI networks, including advanced congestion management, packet-spraying techniques, and link failover. But its important to note these features aren't unique to Cisco, and Nvidia and Broadcom have both announced similarly capable switches in recent years.
例如,我们去年夏天谈到过的思科 Silicon One G200 交换机 ASIC 就具有许多有利于人工智能网络的功能,包括高级拥塞管理、数据包喷洒技术和链路故障转移。但需要注意的是,这些功能并非思科独有,Nvidia 和博通(Broadcom)近年来也推出了类似功能的交换机。
Dell'Oro predicts Ethernet's role in AI networks to capture about 20 points of revenue share by 2027. One of the reasons for this is the industry's familiarity with Ethernet. While AI deployments may still require specific tuning, enterprises already know how to deploy and manage Ethernet infrastructure.
Dell'Oro 预测,到 2027 年,以太网在人工智能网络中的作用将占据约 20% 的收入份额。其中一个原因是业界熟悉以太网。人工智能部署可能仍然需要做一些特定的调整,但企业已经知道如何部署和管理以太网基础设施。
This fact alone makes collaborations with networking vendors like Cisco an attractive prospect for Nvidia. While it may cut into sales of Nvidia's own InfiniBand or Spectrum Ethernet switches, the pay off is the ability to put more GPUs into the hands of enterprises that might otherwise have balked at the prospect of deploying an entirely separate network stack.
对 Nvidia 来说仅这一点就使得与思科等网络厂商的合作是一个有吸引力的前景。虽然这可能会减少 Nvidia 自家 InfiniBand 或 Spectrum 以太网交换机的销售额,但回报是能够将更多 GPU 交付到本来可能对部署完全独立的网络堆栈持怀疑态度的企业。
### Cisco plays the enterprise AI angle
思科的企业人工智能视角
To support these efforts, Cisco and Nvidia have rolling out reference designs and systems, which aim to ensure compatibility and help to address knowledge gaps for deploying networking, storage, and compute infrastructure in support of their AI deployments.
为了支持这些努力,思科和 Nvidia 推出了参考设计和系统,旨在确保兼容性,并帮助解决部署网络、存储和计算基础设施方面的知识差距,以支持其人工智能部署。
These reference designs target platforms that enterprises are likely to have already invested in, including kit from Pure Storage, NetApp, and Red Hat. Unsurprisingly they also serve to push Cisco's GPU accelerated systems. These include reference designs and automation scripts for applying its FlexPod and FlashStack frameworks to AI inferencing workloads. Inferencing, particularly on small domain specific models, are expected by many to make up the bulk of enterprise AI deployments since they're relatively frugal to run and train.
这些参考设计以企业可能已经投资的平台为目标,包括来自 Pure Storage、NetApp 和 Red Hat 的套件。参考设计还有助于推动思科的 GPU 加速系统。其中包括将旗下的 FlexPod 和 FlashStack 框架应用于人工智能推理工作负载的参考设计和自动化脚本。许多人预计推理(尤其是小型特定领域模型的推理)将成为企业人工智能部署的主要部分,因为其运行和训练成本相对较低。
The FlashStack AI Cisco Verified Design (CVD) is essentially a playbook for how to deploy Cisco's networking and GPU-accelerated UCS systems alongside Pure Storage's flash storage arrays. The FlexPod AI (CVD), meanwhile, appears to follow a similar pattern, but swaps Pure for NetApp's storage platform. Cisco says these will be ready to roll out later this month, with more Nvidia-backed CVDs coming in the future.
FlashStack AI Cisco Verified Design (CVD) 是一个将思科的网络和 GPU 加速 UCS 系统与 Pure Storage 的闪存阵列一起部署的指南。而 FlexPod AI(CVD)似乎也遵循了类似的模式,只是将 Pure 换成了 NetApp 的存储平台。思科表示,这些产品将于本月晚些时候推出,未来还将推出更多由 Nvidia 支持的 CVD。
Speaking of Cisco's UCS compute platform, the networking scheme has also rolled out an edge-focused version of its X-Series blade systems which can be equipped with Nvidia's latest GPUs.
提一下思科的 UCS 计算平台,该网络方案还推出了一款专注于边缘的X系列刀片系统,可配备Nvidia的最新GPU。
The X Direct chassis features eight slots that can be populated with a combination of dual or quad-socket compute blades, or PCIe expansion nodes for GPU compute. Additional X-Fabric modules can also be used to expand the system's GPU capacity.
X Direct机箱有8个插槽,可配置双插槽或四插槽计算刀片组合,或用于GPU计算的PCIe扩展节点。额外的 X-Fabric 模块也可用于扩展系统的 GPU 容量。
However, it's worth noting that unlike many of the GPU nodes we've seen from Supermicro, Dell, HPE, and others, which employ Nvidia's most powerful SXM modules, Cisco's UCS X Direct system only appears to support lower TDP PCIe-based GPUs.
不过,值得注意的是,与Supermicro、Dell、HPE和其他厂商所采用的Nvidia最强大的SXM模块不同,思科的UCS X Direct系统似乎只支持功耗较低的基于PCIe的GPU。
According to the data sheet, each server can be equipped with up to six compact GPUs per server, or up to two dual-slot, full- length, full-height GPUs.
根据UCS X Direct数据表,每台服务器最多可配备六个紧凑型 GPU,或最多两个双插槽、全长、全高 GPU。
This will likely prove limiting for those looking to run massive large language models consuming hundreds of gigabytes of GPU memory. However, it's probably more than adequate for running smaller inference workloads, for things like data preprocessing at the edge.
这对那些希望运行消耗数百千兆字节 GPU 内存的大型语言模型的用户来说可能是个限制。不过,在运行较小的推理工作负载时,如边缘数据预处理,这可能已经足够了。
Cisco is targeting the platform at manufacturing, healthcare, and those running small datacenters. ®
思科该平台的定位是制造业、医疗保健业和运行小型数据中心的企业。